Using Customer Sentiment Analysis to Understand Retail Customer Behavior
Discover how retail sentiment analysis enables brands to move beyond surface-level metrics and understand what customers truly feel across reviews, social, chat, and support touchpoints.

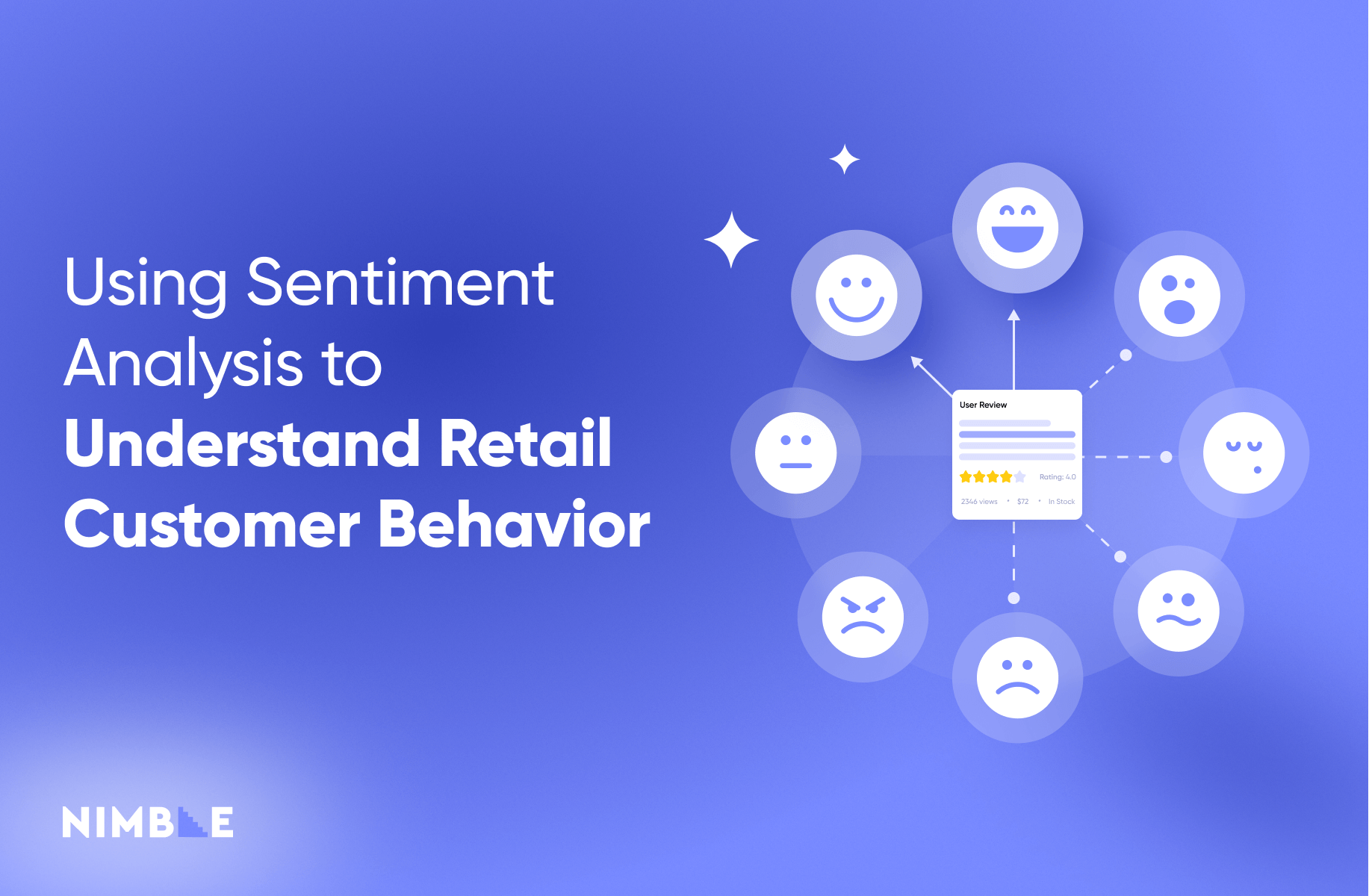
Understanding what your customers feel has never been more critical, or harder to capture.
With conversations happening across dozens of channels at once, manual tracking is too slow, too fragmented, and misses the emotional cues that shape customer behavior.
Customer sentiment analysis tools decode these emotions at scale, analyzing reviews, social posts, and support interactions to reveal real-time insights into shifting moods and brand perception. Retailers can respond faster, adjust messaging mid-campaign, and make sharper, data-driven decisions.
In this article, we explore how platforms like Nimble transform sentiment tracking from manual guesswork into a scalable, always-on strategy.
Key Takeaways
- Sentiment analysis helps retailers track customer emotions, trends, and brand perception.
- AI and automation eliminate manual tracking, enabling real-time, data-driven decisions.
- Retailers can use sentiment insights to refine marketing, improve engagement, and boost sales.
- Automated data collection platforms like Nimbleway make sentiment tracking scalable and efficient.
What is Sentiment Analysis and Why Does It Matter in Retail?
Sentiment analysis is the process of using natural language processing (NLP), machine learning (ML), and artificial intelligence (AI) to interpret the emotions and opinions expressed in text, audio, or social media data. Modern sentiment analysis goes beyond counting positive or negative words. Advanced models analyze context, tone, phrasing, and even irony or sarcasm to accurately classify sentiment at scale.
For retailers, this means turning scattered customer conversations into structured emotional insights. These conversations include reviews, surveys, support transcripts, chat logs, and social media mentions.
The technical backbone involves:
- Data Collection: Pulling structured and unstructured customer data from multiple channels.
- Data Cleaning and Preprocessing: Removing noise (like irrelevant symbols or filler words) to improve model accuracy.
- Sentiment Classification: Using AI models trained on vast datasets to tag each piece of text as positive, negative, neutral, or even nuanced categories like excitement, frustration, or skepticism.
- Scoring and Trend Detection: Assigning sentiment scores to track changes over time and detect emerging patterns.
What makes this critical for retail is not just the analysis itself, but how fast and how usefully it connects to action.
Sentiment insights enable brands to:
- Adjust messaging in real time, such as toning down promotional messaging during moments of public frustration or amplifying successful campaigns.
- Refine product strategies by identifying recurring praise or complaints.
- Proactively resolve customer issues before they escalate to churn or public backlash.
- Enhance personalization, tailoring offers and communications based on customer sentiment trends.
In an industry where consumer preferences shift rapidly, relying on quarterly reports or manual social listening is no longer enough. Automated sentiment analysis bridges the gap between raw data and real-time decisions.
How Retailers Use Sentiment Analysis to Improve Marketing Strategies
Customers don’t wait weeks for brands to react. They expect responsiveness in real time. Using sentiment analysis, marketers can monitor customer emotions dynamically and use those insights to sharpen campaigns, mitigate risks, and seize opportunities.
1. Tracking Customer Emotions in Real-Time to Detect Shifts in Brand Perception
With AI-powered sentiment models continuously parsing live data streams from sources like social media, support chats, reviews, and even voice calls, retailers can detect early signals of shifting customer sentiment.
This kind of analysis ensures brands stay ahead of public perception, rather than scrambling to repair it after the fact.
2. Enhancing Ad Targeting and Personalization
Sentiment data brings a deeper layer of intelligence to audience segmentation. Instead of relying solely on demographic or behavioral data, retailers can now layer in emotional context.
Imagine dynamically adjusting your ad creatives and copy based on detected moods: excitement around a product launch prompts bold, celebratory messaging, while a more cautious sentiment shift might trigger trust-building narratives and reassurances around quality or service.
Sentiment-informed targeting increases relevance and improves conversion rates by speaking directly to how customers feel in the moment.
3. Spotting Emerging Product Trends and Risks
Customer sentiment tracking can forecast future demand or problems. By monitoring recurring themes in sentiment analysis outputs, retailers can spot early signals of a rising product trend, or risks like increasing dissatisfaction.
For instance, if social listening detects growing enthusiasm for sustainable materials or frustration over packaging waste, brands can adapt campaigns and product development roadmaps accordingly, staying ahead of both consumer demand and potential PR pitfalls.
4. Optimizing Brand Messaging and Reputation Management
Sentiment analysis empowers brands to maintain precise messaging at scale. AI tools flag tone-deaf communications early, highlight positive brand associations to amplify, and ensure alignment across customer touchpoints.
In practice, this means:
- Rapidly identifying which messaging resonates best in current market conditions.
- Mitigating reputational risks by swiftly responding to negative sentiment spikes.
- Maintaining brand consistency across regions by understanding cultural sentiment nuances.
In an environment where brand perception can shift overnight, having automated sentiment monitoring in place transforms reputation management from reactive to proactive.
Challenges of Sentiment Analysis and How Automation Solves Them
While customer sentiment analysis offers powerful insights, many retailers still struggle to make it work at scale. Manual processes and fragmented data sources create delays and blind spots, limiting the potential of sentiment-driven strategies.
The Problem with Manual Data Collection
Manually gathering customer feedback from emails, reviews, social media, and support channels is time-intensive and leads to incomplete data. Human analysts can only process so much data, and by the time insights are compiled, customer sentiment may have already shifted.
Retailers relying on static, manual reports risk falling behind fast-moving customer expectations. Moments of frustration or excitement that could have guided campaigns or support interventions slip through the cracks.
Fragmented Data Across Platforms
Modern retail ecosystems span dozens of touchpoints, from online storefronts and chatbots to call centers and third-party review sites. Without centralized data aggregation, sentiment insights remain siloed, preventing brands from seeing the full emotional landscape of their customer base.
This fragmentation leads to incomplete or misleading sentiment readings. A product might be trending positively on social media, while unresolved support tickets tell a very different story. Without a unified view, brands can misinterpret customer mood and miss critical signals.
How Automation Changes the Game
Effective sentiment analysis requires automating three critical stages: data collection, data processing, and analysis. Without automation, each step creates friction that delays insights and limits scale.
Automated sentiment analysis tools like Nimble’s solve these challenges by delivering a fully integrated, real-time workflow:
1. Automated Data Collection
Manual collection is slow and fragmented. Nimble automatically gathers sentiment signals from across social media, customer reviews, support transcripts, call logs, and more—ensuring a live, unified feed of customer emotion.
2. Automated Data Processing
Raw data is cleaned, normalized, and structured using AI. This includes noise filtering, standardizing language (like slang and emojis), and parsing text for better context—laying the foundation for accurate analysis.
3. Real-Time Sentiment Analysis
Advanced NLP models classify and score sentiment across thousands of data points. Automation detects not just polarity, but nuances like frustration, excitement, or confusion. It also highlights emerging trends in real time.
By automating this end-to-end workflow, Nimble empowers retailers to act fast, personalize more effectively, and stay ahead of shifts in customer mood.
Nimble: Sentiment Analysis at Retail Speed
Nimble’s Online Pipelines take sentiment analysis beyond dashboards, automating the entire workflow from data collection to real-time action. For retail executives, this means immediate access to the emotional signals shaping customer behavior, with no manual work or guesswork required.
Instead of stitching together tools, retailers get a single pipeline that:
- Continuously collects customer sentiment from social media, reviews, support chats, and more.
- Cleans and standardizes text data for higher model accuracy across formats and languages.
- Classifies and scores sentiment using built-in NLP models that detect emotion and nuance.
- Surfaces real-time trends and pushes insights directly into CRMs, ad tools, or alerts.
With Nimble, retailers move from lagging reports to live emotional intelligence: ready to act, personalize, and compete in real time.
Best Practices for Implementing Sentiment Analysis in Retail
To deliver actionable insights that genuinely influence marketing and branding strategies, retailers need to apply a methodical, technically sound approach. Let’s break it down.
1. Capture Multi-Channel Sentiment Data at Scale
To build a holistic view of customer emotion, retailers must cast a wide net across all customer touchpoints. This involves aggregating structured and unstructured data from multiple platforms, including:
- Social Media Platforms: Real-time emotional feedback through posts, comments, hashtags, and mentions.
- Online Reviews and Ratings: Rich, descriptive opinions on products and services.
- Direct Customer Feedback: Survey responses, NPS (Net Promoter Score) data, and customer satisfaction surveys.
- Customer Service Interactions: Transcripts from live chats, emails, and call recordings (voice analysis adds an extra layer of emotional depth).
- Third-Party Forums and Communities: Unfiltered sentiment from niche audiences (e.g., Reddit threads, specialized forums).
Use timestamping and source-tagging during data collection. This allows for chronological sentiment tracking and cross-channel comparison.
2. Analyze and Categorize Sentiment with Precision
Once collected, raw data needs to be cleaned and preprocessed to improve model accuracy. Key steps include:
- Noise Reduction: Strip out irrelevant data like filler words, spam, or boilerplate signatures.
- Language Normalization: Standardize slang, abbreviations, and emojis (e.g., 😊 = positive; 😠 = negative).
- Tokenization and Parsing: Break down text into analyzable units (tokens), parse sentence structure, and contextual cues.
Modern sentiment models move beyond simplistic positive/negative/neutral classifications. Advanced NLP techniques can do things like:
- Aspect-Based Sentiment Analysis: Break down multi-topic reviews to analyze sentiment per product feature (e.g., "Great price but slow shipping").
- Emotion Classification: Detect specific emotions (anger, joy, frustration) instead of broad categories.
- Contextual Embeddings: Understand word meaning based on context, improving interpretation of nuanced language like sarcasm or mixed sentiments.
Sentiment scoring frameworks then quantify intensity, not just polarity, providing weighted scores (e.g., -0.8 for strong negative, +0.9 for highly positive) that help prioritize actions.
Continuously retrain models with fresh, domain-specific data to reduce drift and improve accuracy over time.
3. Operationalize Sentiment Insights in Marketing and Branding
Collecting and categorizing sentiment is only half the equation. True value comes from integrating insights directly into retail decision-making workflows.
Key tactics include:
- Dynamic Campaign Optimization: Feed real-time sentiment signals into ad platforms (Google Ads, Meta) to adjust targeting, creative, and bidding strategies based on live audience mood.
- Product Strategy Alignment: Use sentiment trend analysis to prioritize feature updates or identify underperforming products early.
- Reputation Management Workflows: Trigger automated alerts when sentiment drops below defined thresholds, allowing rapid response from PR or social teams.
- Customer Segmentation Refinement: Enrich customer profiles with sentiment scores for hyper-targeted outreach. For example, retarget satisfied customers with loyalty offers, or offer win-back incentives to disenchanted segments.
Integrate sentiment dashboards into your marketing tech stack (CRM, CDP, or BI tools) to ensure insights are accessible across teams, from marketing to merchandising to customer support.

How Nimble Powers Retail Sentiment Analysis at Scale
For organizations looking to unlock the full potential of retail sentiment analysis, speed and scale are non-negotiable. Nimble for Retail’s automated data collection and sentiment analysis infrastructure is purpose-built to meet these demands, delivering accurate, real-time insights without the manual overhead.
Automated, Comprehensive Data Collection
Nimble’s Online Pipelines automates sentiment data collection across every critical customer touchpoint. Sources include:
- Social Platforms: Posts, comments, and real-time reactions from Twitter, Reddit, TikTok, and more.
- E-commerce Sites: Product reviews and ratings from marketplaces and brand-owned stores.
- Customer Support Channels: Transcripts from live chats, email threads, and voice call metadata.
- Third-Party Forums and Communities: Niche conversations in places like Discord, Quora, and industry-specific message boards.
Online Pipelines consolidates all of this into a single, continuously updated stream. Built-in data normalization ensures consistency across languages, formats, and platforms, delivering a true 360° view of customer sentiment. Nimble’s distributed infrastructure supports large-scale collection while maintaining compliance and respecting platform guidelines.
Real-Time Sentiment Tracking for Agile Decision-Making
Nimble’s real-time processing engine applies advanced NLP and machine learning models to classify, score, and surface actionable sentiment insights the moment they appear. Whether it’s a surge of positive reactions to a new product drop or early signs of frustration over supply chain delays, brands receive immediate visibility and can act fast.
Key benefits include:
- Instant Trend Detection: Identify sentiment spikes tied to campaigns, events, or market changes.
- Automated Alerting: Get notified when sentiment breaches defined thresholds (e.g., sustained negative sentiment on a key product).
- Dynamic Data Routing: Push sentiment data directly into CRM, ad platforms, or analytics tools for automated response flows.
From Insight to Impact: Real-World Retail Applications
With a scalable retail sentiment analysis infrastructure, businesses can move from passive observation to proactive strategy. Here’s how leading brands are applying sentiment insights in practice:
Optimizing Live Campaigns
Scenario: A sportswear brand launches a product and monitors real-time sentiment across social media. Early reactions highlight excitement about the design but complaints about sizing. Marketing teams adjust ads to promote broader size availability, capitalizing on positive buzz while addressing frustrations.
Product Feedback Loops
Scenario: A beauty retailer uses Nimble to monitor product reviews and customer service chats. Analysis flags recurring mentions of packaging. The insights are routed directly to the product development team, prompting a packaging redesign before the issue scales.
Crisis Management
Scenario: A fashion retailer faces a sudden backlash over delayed holiday shipments. Nimble’s real-time alert system detects a spike in negative sentiment across social channels and customer service interactions. The brand quickly deploys a proactive apology campaign, offering expedited shipping for pending orders.
Customer Retention Programs
Scenario: A retailer segments its customer base by sentiment history, identifying a group of first-time buyers expressing mild dissatisfaction with shipping times. The marketing team launches a retention campaign offering discounts on accessories, successfully turning at-risk customers into repeat purchasers.
Conclusion: Make Every Customer Signal Count
AI-powered retail sentiment analysis gives retailers an edge: real-time visibility into customer emotions, faster reactions to shifting trends, and sharper marketing decisions. Automation makes this process scalable, turning scattered data into clear, actionable insights.
With Nimble, brands move from slow, manual tracking to instant sentiment clarity, and smarter strategies that keep pace with customer expectations.
FAQ
Answers to frequently asked questions