How Alternative Data & AI Breakthroughs in Finance Can Revolutionize Retail & CPG
Learn how advancements in alt data & AI in the financial industry can be applied to retail & CPG to drive growth, profit, and efficiency.
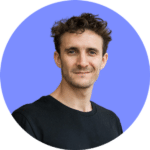
.png)
Over the past decade, the financial services industry has undergone a significant transformation driven by the adoption of alternative data and artificial intelligence (AI). Hedge funds, investment banks, and asset managers have leveraged these tools to gain a competitive edge by harnessing vast amounts of web data to inform trading strategies and investment decisions.
This new approach to data analytics is now converging with the enterprise data world, creating an unprecedented opportunity for the retail and Consumer Packaged Goods (CPG) sectors. As AI becomes more accessible and data analytics tools advance, retail and CPG companies stand on the cusp of a revolution.
This article explores how recent developments in alternative data and AI in the finance industry could be applied to the world retail and CPG. By adopting strategies honed in the financial industry, retail sectors can accelerate growth, enhance customer experiences, and drive innovation.
The Rise of Alternative Data in Finance
In the past ten years, the financial services industry has embraced “alternative data”—a term that encompasses all non-traditional data sources and data types that provide unique insights beyond conventional financial statements and market data.
What Is Alternative Data?
Alternative data used by the financial industry includes:
- Web Scraped Data: Information gathered from websites, social media, and online forums.
- Satellite Imagery: Used to estimate retail foot traffic or agricultural yields.
- Geolocation Data: Mobile device data to track consumer movements and behaviors.
- Transactional Data: Credit card transactions providing real-time spending patterns.
Technological advancements in analyzing, collecting, and interpreting data are also cast under the umbrella of “alternative data”, and are heavily used by the financial industry. These advancements include:
- Natural Language Processing (NLP): AI models that interpret human language have become crucial in analyzing textual data from the web.
- Machine Learning Algorithms: Capable of identifying patterns and making predictions based on large datasets.
- Real-Time Data Processing: Technologies that allow for immediate analysis of incoming data streams.
How Finance Budgets Have Shifted to Prioritize Data
Due to the effectiveness of these novel data streams, the spending on alternative data in the financial sector has seen exponential growth.
According to industry reports, financial firms' budgets for purchasing alternative data have increased by over 30% annually in the past few years. Moreover, significant are allocated to AI and machine learning platforms to handle complex data analysis, with a heavy focus on high-return data investments that can provide a competitive advantage.
This shift reflects a mature data process where the value of data is well-recognized, and investments are strategically directed toward data sources and technologies that drive results.
How Alternative Data and AI is Used In Financial Services
Financial institutions have invested heavily in data acquisition and analytics platforms to collect, process, and interpret alternative data. AI and machine learning models have become integral in extracting actionable insights, enabling firms to:
Predict Market Movements
- Example: Using consumer sentiment analysis from social media and news sources to predict stock price fluctuations.
- Impact: Investors gain an edge by anticipating market shifts before they are reflected in stock prices.
Assess Company Performance
- Example: Analyzing supply chain data for signs of delays or disruptions to assess the financial health of companies.
- Impact: Enables early detection of operational issues, allowing for more informed investment decisions.
Optimize Trading Strategies
- Example: Leveraging real-time data feeds to implement algorithmic trading strategies that adjust instantly to market changes.
- Impact: Traders can execute faster and more accurate trades, optimizing profits in volatile markets.
Collect and Analyze of Economic Indicators
- Example: Using web search trends to predict unemployment rates or consumer spending.
- Impact: Allows for more timely and accurate economic forecasts.
Enable Better Risk Management
- Example: Monitoring web data for signs of geopolitical events or natural disasters that could affect markets.
- Impact: Enables proactive risk mitigation strategies.
Translating Financial Strategies to Retail and CPG
Relevance and Applicability
The sophisticated use of alternative data and AI in finance offers valuable lessons for retail and CPG companies:
- Customer Insights: Just as investors predict market movements, retailers can anticipate consumer behaviors.
- Supply Chain Optimization: Monitoring web data can provide early warnings about disruptions.
- Competitive Analysis: Keeping track of competitors' online activities to inform strategic decisions.
Case Study: Using Satellite Imagery Techniques for Inventory
As a hypothetical example, say a hedge fund uses satellite imagery to estimate the number of cars in retail parking lots, predicting quarterly earnings. A retail company could adopt a similar approach:
- In-Store Analytics: Use foot traffic data to optimize store layouts and staffing.
- Market Demand Forecasting: Analyze online search trends for product categories to adjust inventory levels.
Additional Use Cases for Alt Data and AI in Retail and CPG
Often, the data and AI technology finance companies utlize are not directly transferable to retail and CPG. Nevertheless, the alternative data technologies the financial industry has helped developed have a myriad of use cases that are unique to retail and CPG.
A few ways AI and alternative data can directly impact the retail and CPG industry include:
1. Personalization at Scale
- Dynamic Pricing: Adjusting prices in real-time based on demand and inventory.
- Customized Marketing: Tailoring promotions to individual customer preferences.
2. Enhanced Supply Chain Management
- Predictive Analytics: Anticipating demand fluctuations to optimize inventory.
- Real-Time Tracking: Monitoring shipments to improve logistics efficiency.
3. Product Development
- Trend Analysis: Identifying emerging consumer trends through social media and search data.
- Feedback Integration: Incorporating customer reviews into product improvements.
Technological Enablers
Technologies that are set to positively impact the retail and CPG spaces include:
- Machine Learning Platforms: Tools like Databricks facilitate the development and deployment of machine learning models at scale.
- AI Models and APIs: Services from OpenAI enable companies to integrate advanced AI capabilities without building models from scratch.
- Vector Databases: Pinecone and similar technologies enhance the performance of AI applications that require similarity search and real-time recommendations.
- Workflow Automation Tools: Platforms like Nimble streamline the creation and management of AI workflows, reducing complexity and accelerating deployment.
Challenges and Considerations
While these technologies and strategies will be helpful to improve profitability and efficiency, they also come with potential challenges:
- Data Privacy: Navigating regulations like GDPR and ensuring ethical data use.
- Talent Acquisition: Building teams skilled in data science and AI.
- Technology Integration: Updating legacy systems to handle modern data processing demands.
The Oncoming Wave: How Retail and CPG Budgets Are Already Prioritizing Alt Data and AI
On the whole, retail and CPG companies are already recognizing the need to invest in data acquisition and analytics, and are prioritizing large portions of their budget toward the fields of alt data and AI.
Retail and CPG budgets are increasing to purchase alternative data sources, including web data, social media analytics, and IoT sensor data. Investments in AI platforms and analytics tools are growing rapidly to process and interpret data more effectively, and larger allocation of funds are going towards tools that streamline AI workflow creation and deployment.
Factors Driving Budget Changes
The reason the retail and CPG industries are prioritizing data so much is multi-faceted. For most companies, reasons include:
- Competitive Pressure: E-commerce giants leveraging data analytics are setting new industry standards.
- Customer Expectations: Personalized experiences require detailed customer insights.
- Operational Efficiency: Data-driven decision-making leads to cost savings and improved margins.
- Accessibility of Advanced Tools: The availability of platforms like Nimble makes advanced AI more accessible and cost-effective.
How Retail and CPG Companies Can Prepare for the Data-Driven Future
Here are a few steps you can take as a retail or CPG company to position yourself advantageously in the face of the oncoming data revolution.
1. Embrace a Data Culture
- Encourage data-driven decision-making across all organizational levels.
- Invest in training programs to enhance data literacy.
2. Invest in Infrastructure
- Upgrade IT systems to support big data and AI applications.
- Leverage cloud technologies for scalability.
3. Form Strategic Partnerships
- Collaborate with data providers and technology firms specializing in AI and analytics.
- Consider alliances with companies experienced in alternative data, like those in the financial sector.
4. Focus on Data Quality
- Implement robust data governance practices.
- Ensure data collected is accurate, relevant, and timely.
5. Innovate Continuously
- Pilot AI initiatives in various business areas.
- Stay abreast of emerging technologies and industry best practices.
6. Leverage Workflow Automations
- Utilize platforms like Nimble to streamline AI workflows and reduce time-to-value.
- Automate repetitive tasks to allow teams to focus on strategic initiatives.
The Role of Leadership
Executive support is crucial in driving the transformation as well. CPG and retail leaders must prioritize the following traits:
- Vision Setting: Leaders must articulate a clear vision for leveraging data and AI.
- Resource Allocation: Prioritize funding for data initiatives.
- Risk Management: Address concerns related to data security and compliance proactively.
- Championing Innovation: Encourage a culture that embraces change and continuous improvement.
The Technical Side of Integration
So how exactly can you take advancements in AI and data–whether they’re sourced in the finance industry or not—and apply them to your retail and CPG businesses? Here’s a quick overview of some tools, platforms, and steps you can take.
Step 1: How to Leverage Modern Tools and Platforms
Integrating alternative data and AI into retail and CPG operations requires a robust technical infrastructure. Key technologies and platforms facilitating this integration include:
1. Databricks: Unified Data Analytics Platform
- Overview: Databricks offers a cloud-based platform that integrates data engineering, machine learning, and analytics.
- Benefits:
- Scalability: Handle large volumes of structured and unstructured data.
- Collaboration: Enable data scientists and engineers to work together seamlessly.
- Real-Time Processing: Support streaming data for immediate insights.
- Retail/CPG Application Example:
- Retailers can use Databricks to process transaction data and web analytics in real-time, feeding into AI models for dynamic pricing strategies.
2. OpenAI: Advanced AI Models
- Overview: OpenAI develops state-of-the-art AI models, including GPT-3 and GPT-4, which excel in natural language understanding.
- Benefits:
- Language Processing: Analyze customer reviews, social media, and other textual data.
- Chatbots and Virtual Assistants: Enhance customer service with AI-driven interactions.
- Content Generation: Automate personalized marketing content creation.
- Retail/CPG Application Example:
- A CPG company can use OpenAI models to analyze consumer sentiment across social media platforms, informing product development and marketing strategies.
3. Pinecone: Vector Database for Machine Learning
- Overview: Pinecone provides a vector database optimized for machine learning applications, particularly useful for similarity search and recommendation systems.
- Benefits:
- High Performance: Efficiently handle large-scale machine learning workloads.
- Ease of Integration: API-based access simplifies incorporation into existing systems.
- Real-Time Recommendations: Power recommendation engines with low latency.
- Retail/CPG Application Example:
- An e-commerce retailer can leverage Pinecone to enhance their product recommendation system, improving personalization and increasing sales.
Step 2: How to Create AI and Alt Data Workflows
Efficiently managing and orchestrating AI-driven processes is crucial for maximizing the value of data integration. Workflow automation platforms enable businesses to create, deploy, and scale AI workflows with minimal effort.
Nimble's AI Workflows
Nimble provides a low-code, serverless solution for integrating complex AI and data tasks into custom workflows through our managed pipelines. From data collection and transformation to activating insights with AI-driven outputs, Nimble simplifies the entire process, ensuring seamless integration with large-scale AI models and data environments.
Key Features of Nimble’s AI Workflows
1. Build Custom AI Workflows
- Create and configure AI-driven workflows using a user-friendly interface.
- Integrate data from multiple sources (JSON, SQL, CSV).
- Run data transformations, including validation, cleaning, and formatting using semantic agents.
- Leverage AI-driven matching and classification models through integrations with platforms like Hugging Face and OpenAI.
2. Effortless AI Model Integration & Orchestration
- Integrate state-of-the-art models, databases, and storage solutions.
- Select models from Hugging Face or OpenAI using API keys.
- Incorporate advanced functions like vector search, embedding generation, and entity matching.
- Orchestrate AI workflows with low latency and scalability.
3. Publish, Manage, and Monitor AI Pipelines
- Version control for workflows with seamless deployment to staging or production environments.
- Monitor AI performance, tracking metrics like accuracy and response times.
- Deploy AI workflows at scale using serverless API endpoints.
Benefits of Nimble’s AI Workflows
- No Infrastructure Needed: Serverless architecture allows for building and deploying AI workflows without managing servers.
- AI Orchestration: Coordinate multiple models and APIs to ensure seamless execution of AI-driven workflows.
- Model Flexibility: Integrate pre-trained or custom data science models, running them on ML Ops platforms behind the scenes.
- Scalable Deployment: Effortlessly scale AI workflows to meet operational needs with production-ready deployment.
Example Use Cases of Nimble’s AI Workflows
E-commerce Product Matching
- Data Collection Agent: Gathers product data from multiple retail websites.
- Semantic Matching Agent: Uses a product-matching model via Hugging Face to classify and match items across platforms.
- Post-Processing Agent: Filters products based on availability and price thresholds.
- Answer Agent: Generates reports and sends alerts for unmatched products, delivering results via an API endpoint.
Financial Sentiment Analysis
- Data Collection Agent: Collects real-time financial news articles and market data.
- Semantic Analysis Agent: Utilizes a pre-trained language model to analyze sentiment and classify data based on financial indicators.
- Reporting Agent: Delivers daily sentiment reports to business intelligence dashboards.
Step 3: How to Implement Integration Strategies
Here are some tools and platforms you can use to organize, analyze, and manage your data process.
- Data Integration Platforms: Utilize tools like Apache Kafka or AWS Glue to integrate disparate data sources into a unified data lake.
- Cloud Infrastructure: Leverage cloud services (e.g., AWS, Azure, Google Cloud) for scalable storage and computing resources.
- APIs and Microservices: Employ API-driven architectures to enable modular, scalable system designs.
- Security and Compliance: Implement robust security measures, including encryption and access controls, to protect sensitive data and comply with regulations like GDPR.
Step 4: Troubleshooting Common Challenges and Solutions
Here are a few common challenges when collecting, analyzing, and exploring data, as well as their solutions.
1. Data Quality and Consistency
- Challenge: Integrating data from multiple sources can lead to inconsistencies.
- Solution: Implement data cleansing processes and use data validation tools.
2. Legacy Systems Integrations
- Challenge: Older systems may not easily interface with modern platforms.
- Solution: Use middleware or data integration platforms to bridge the gap.
3. Talent and Expertise
- Challenge: Shortage of skilled professionals in data science and AI.
- Solution: Invest in training, hire specialists, or partner with tech firms.
Conclusion: The Data Revolution Is Here—Make Sure You’re Prepared
As AI technology becomes more democratized and data more accessible, the barriers to entry are lowering. Organizations that act now to integrate these advanced data strategies and workflow automation tools will position themselves at the forefront of their industries, ready to meet the challenges of tomorrow's marketplace.
By learning from the financial industry's mature data processes and leveraging advanced technologies like Databricks, OpenAI, Pinecone, and Nimble's AI Workflows, retail and CPG companies can accelerate their evolution and implement them to achieve better business operations and drive innovation, efficiency, and growth.
The journey requires commitment, investment, and a willingness to embrace change. However, the potential rewards—enhanced customer experiences, optimized operations, and sustained competitive advantage—make it a strategic imperative.
If you’d like to learn more about how you can use Nimble’s tools to adopt cutting-edge data processes and accelerate your business growth, get in touch with us today.
FAQ
Answers to frequently asked questions